How to introduce AI in your organization
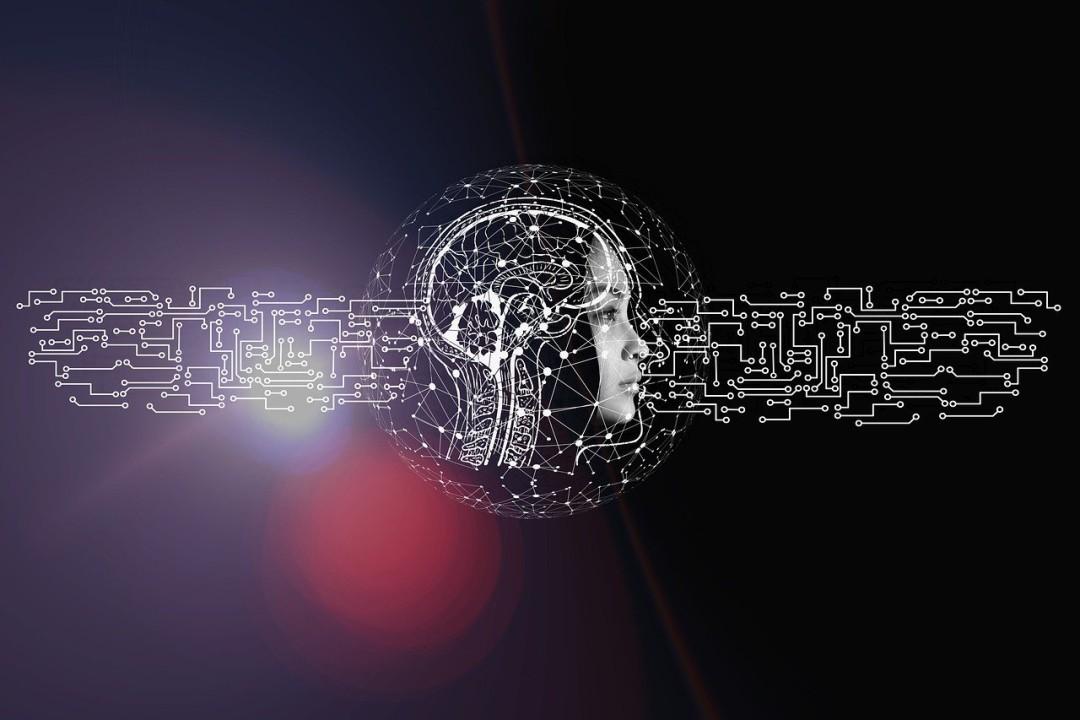
AI
Digital Transformation
Machine Learning
Why is it so difficult to introduce AI in Companies?
It’s our fault that it seems difficult to introduce AI in organisations and companies. We (the AI industry) mythicise it.
AI is not magic. What is mostly known as AI today, is actually Machine Learning. Machine Learning is a computation paradigm that allows one to “teach” a computer something, based on examples.
We don’t teach the computer the theory, we teach it practical examples. And we do that long enough, until we are happy with how well the computer is doing, whenever a new example comes.
That’s it.
So, why is it so difficult? Because data scientists, engineers and the media mythicise this. And because knowledge is power, the experts protect it, because they want to keep their power. Machine Learning is just another discipline of Computer Science.
Translating a business problem to a Machine Learning problem is just another skill that business analysts need to develop.
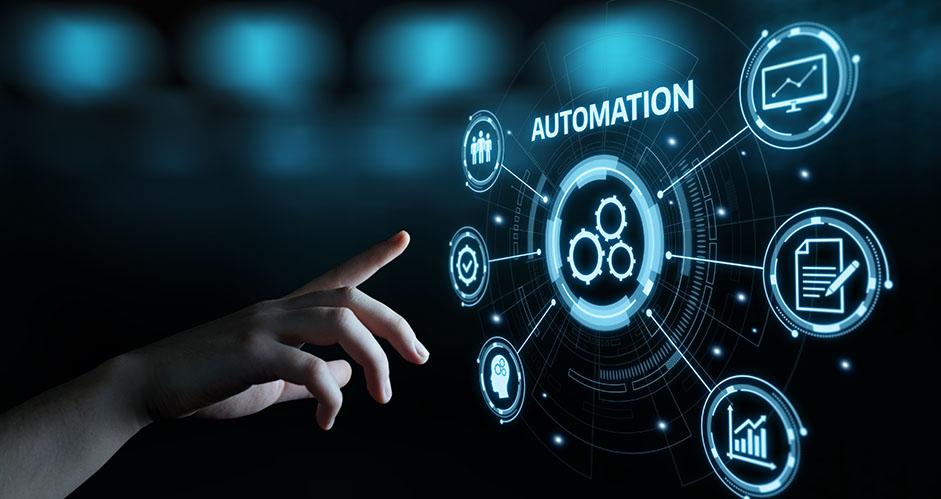
6 Steps to Introduce AI in Organisations
In my experience, it helps to have a process that supports the journey. The following steps are useful in looking at efficiency challenges and identifying opportunities. This is not meant to be a recipe for a holistic vision of the future of your business, but a non-exhaustive list of steps that have proven to be useful in the past.
1. Identifying the business challenges
Just like for many other situations, identifying the biggest opportunities for improvement is the very first step to take in order to introduce AI in any organisation.
- Which areas of your organization are the most inefficient?
- How could you better serve your customers?
- How can you save money?
2. Come up with possible solutions
After identifying the challenges and opportunities, it’s time to brainstorm possible solutions and approaches.
In the manufacturing industry, a typical challenge is to analyse how much energy is spent during the process. After all, energy is often one of the largest costs in production.
- Can I save energy with better data? What if you could make better energy-saving decisions?
- If you were to solve the problem, what would you do, how much effort would it take, and how much money would it cost?
If we focus on other areas, the questions change accordingly. In insurance, for example, typical questions are:
- How much does it cost me to evaluate a claim? How can I make this process more efficient?
3. Set priorities
There will be many ideas of how to increase efficiencies, save money, and introduce new revenue streams out of the second step. So the third step is for each of those cases to be prioritised.
A good exercise is to use an impact vs. effort matrix.
The so-called low hanging fruits are those that have a high impact with a low effort. These are where you should begin with.
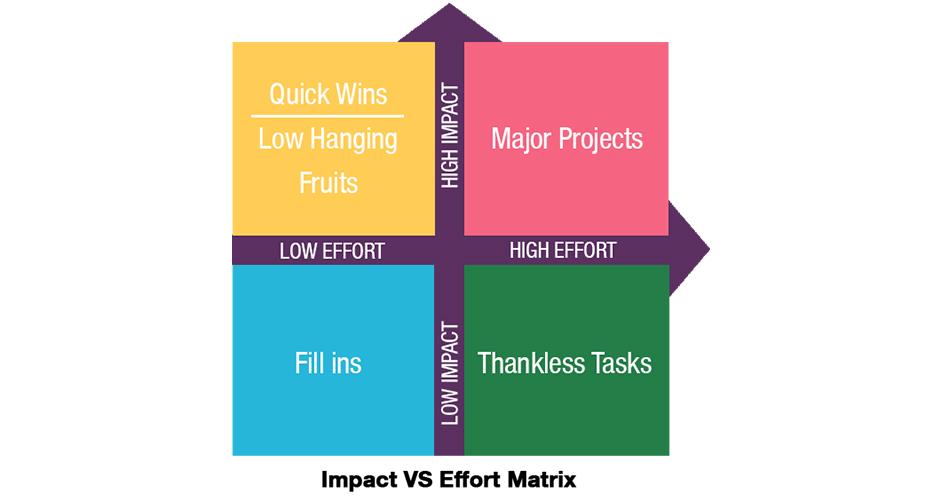
4. Proof of concept (PoC)
In this stage, you take one of those lowest hanging fruits and implement a Proof of Concept. The goal is to prove that the problem can be solved in the way that it was conceived. A better and more accurate estimate can be done of the effort in the cast of involvement in such a case.
5. Minimum Viable Product (Go live)
Once the proof of concept has been proven, the idea is to go live with the solution. A data-driven solution has several stages of implementation:
- Data gathering and integration
- Designing a Data Lake (or a Data Warehouse)
- Developing Predictive and Machine Learning models to bring added value
- Deliver insights in the form of visualizations, alarms, recommendations, or integration with current IT systems and business processes
6. Improve. Improve. Improve.
Going live is not the end of your work. It is only the beginning. You’ll only understand the benefits of the new solution once it’s in your users’ hands.
After that, a continuous improvement process is key. Be sure to save budget for this, since it is as important as the conceptual and project phase.