The Transformation of Customer Service Through Large Language Models
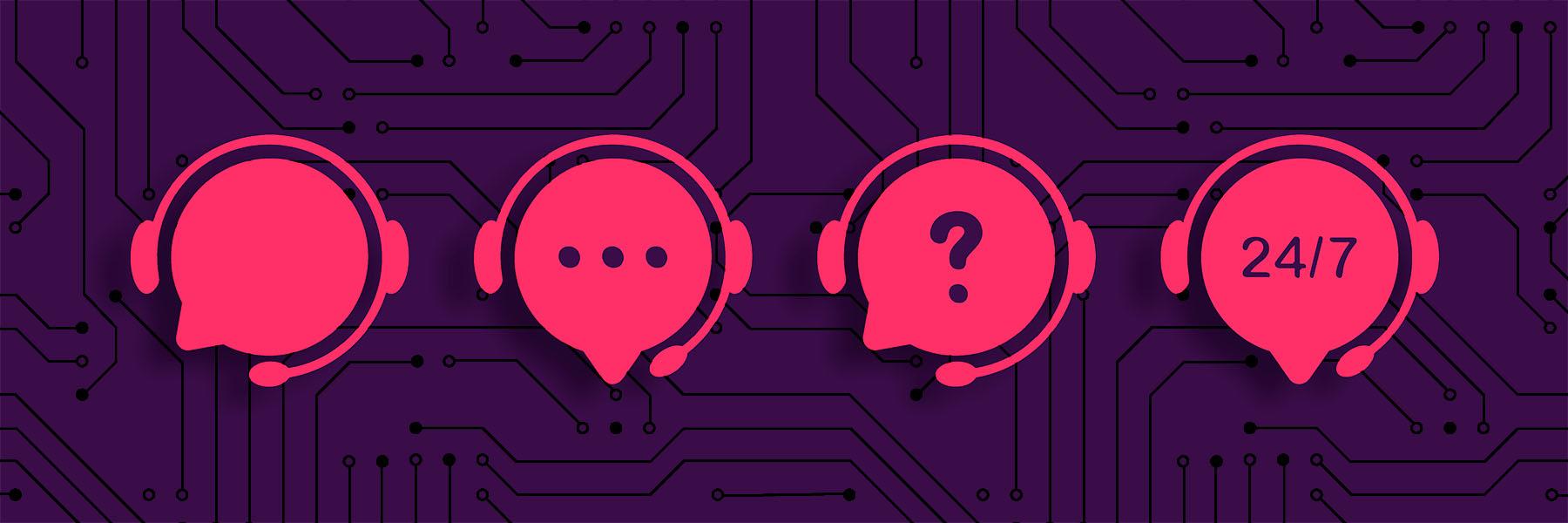
Customer Service
Large Language Models
Chatbots
ChatGPT
No house starts being built from the roof down, so before actually covering how customer service is being transformed by Large Language Models, we should first build the foundations and go over what exactly is customer service.
I’ll also summarize all the technology that is (or can be) used in customer service. That way, you’ll definitely be better prepared to read about what LLMs are, their applications on customer service, effect on existing technology, what to keep in mind or how to get started. And yes, I do cover all that in this article.
What is Customer Service?
Customer service is a central component of any business that deals directly with consumers. It includes addressing customer needs, resolving issues, and ensuring optimal customer satisfaction. Successful customer service can lead to increased customer loyalty, positive word-of-mouth, and ultimately higher profits for the business.
Types of Customer Service Technology
Phone
The symbol of customer service since the early days of the intimidating switchboard call centers. Other channels have come, but that personal touch and real time conversations still remain reassuring for many customers.
Interactive Voice Response (IVR)
IVRs were the first big leap in customer service automation. Giving callers the possibility to choose the reason why they’re calling, just by pressing a button on the phone, allowed centers to really start optimizing their operations.
Live Chat
The massification of the internet brought live chat to customer service, and with it another option for customers combining immediateness and convenience.
Although email does not work in real-time, it does allow for more detailed responses and, probably its most valuable feature, to keep a record of communication.
Messaging Channels
Messaging apps like Facebook Messenger and WhatsApp allow the communication to happen through platforms customers are already familiar with, and use daily, making it also more personal than live chat.
Chatbots
Chatbots promised golden communication, but often turned out to be nothing but brass. But Large Language Models are finally making a fundamental change in this industry, as we will discuss below.
Voicebots
The success of virtual assistants like Alexa or Siri promised a bright future for voicebots. But it has been held back by poor implementations and some technology limitations.
Customer Relationship Management
CRMs, like Salesforce or Hubspot, help businesses manage interactions with customers across the various channels and touchpoints.
Customer Ticketing Systems
These are invaluable to manage and track customers’ inquiries and issues, optimizing operations, increasing accountability and reducing response times.
Customer Service Platforms
Some modern solutions combine several technologies for customer experience and contact centers, like omnichannel routing, predictive engagement, reporting and analytics, call recording and monitoring, among other.
Enter Large Language Models
As we stand on the brink of a new era, Large Language Models (LLMs) like GPT are setting the stage for the next evolution in customer service technology.
LLMs are AI models designed to understand, generate, and respond to human language in a way that is remarkably similar to how humans do. Trained on vast amounts of text data taken from the internet, these models are super-sized, with millions or even billions of parameters. It supports their ability to understand context, nuances, and even the subtleties of language, allowing them to provide more sophisticated and human-like interactions.
In simple terms, an LLM works by predicting the next word in a sentence based on the words it has seen so far. This ability to generate coherent text has greatly improved since 2017, thanks to a type of model architecture called the transformer.
Previously, to get these models to do specific tasks, we had to fine-tune them. But with the latest, larger models, like GPT-4, we can now just give them a detailed prompt and they can do a good job.
But remember, these models learn from the data they are trained on. So, if the data has mistakes or biases, the model will learn those too.
Some well-known LLMs are the GPT series (like GPT-3.5 and GPT-4, which power ChatGPT), LLaMa, PaLM (used in Google Bard), BLOOM, Ernie 3.0 Titan, and Claude.
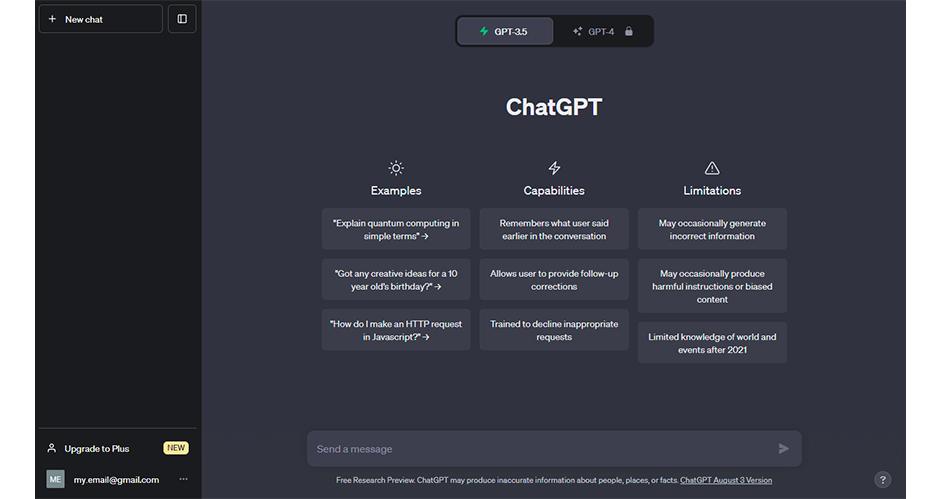
LLMs and Their Application in Customer Service
LLMs have a transformative potential in customer service. By understanding and generating human-like responses, they can handle customer queries and complaints more efficiently and effectively than ever before. They can process complex patterns and nuances in customer interactions, behavior, and preferences to create finely-tuned customer profiles, which then can be leveraged to create tailored product recommendations, enhancing client satisfaction and engagement.
Large Language Models can be incorporated into customer service to, for example:
- Deliver personalized customer service experiences at scale
- Answer queries accurately
- Offer personalized product suggestions
- Provide proactive service
- Perform sentiment analysis, enabling businesses to gain valuable insights into their customer base
- Generate responses to customer queries in a more human-like manner, leading to a superior and seamless customer experience.
Let’s take a look at some key applications of LLMs in customer service in more detail.
Upgrading Chatbots and Voicebots
Classic Chatbots or Voicebots use intent classification to "understand" what the customer is saying. They use a machine learning model to classify a sentence, then use logic (flows, stories, etc) to make decisions about what to do and say next. Some of them keep some context of the conversation and actually know something about the customer. Despite all the efforts to do good conversational design, introduce good logic and make use of context, using chatbots can still be quite frustrating to users. LLMs can help change this.
LLMs can be deployed to take chatbots to the next level, and make them really understand language, as well as keeping a pretty good context. If you know a user, you can give the LLM a prompt (or a prefix) that gives them that context, and help tailor the conversation, offering this way a truly personalized experience to customers.
Unlike traditional chatbots, LLM-based chatbots are able to understand the context and nuances of customer queries, resulting in more accurate and human-like interactions.
By bringing LLMs to the picture, chatbots can finally start seeing real adoption from the majority of customers, and become a real alternative to customer service agents.
But it’s not all sunshine and rainbows. There are risks associated with introducing chatbots in customer or public-facing scenarios. Problems like hallucination (which we cover further down in this article), bias and others, can damage a brand's reputation and even put customers at risk. So, using chatbots for customer facing use cases is still a sensitive topic.
A Super Customer Service Agent
LLMs can revolutionize the way customers and people in general find information. They can be trained to retrieve and present relevant information from vast knowledge bases, thereby answering customer queries promptly and accurately.
This could range from product features and usage instructions to troubleshooting guides and policy details. With an AI-powered knowledge base lookup, customers can find the information they need without sifting through piles of unrelated content, improving their overall service experience.
In a customer service scenario, a customer service agent is not only able to find information much faster than with a classic search engine, but also formulate an appropriate answer in a matter of a few seconds, resulting in a superior customer experience but also dramatically improved productivity. It's like having a customer service agent with superpowers!
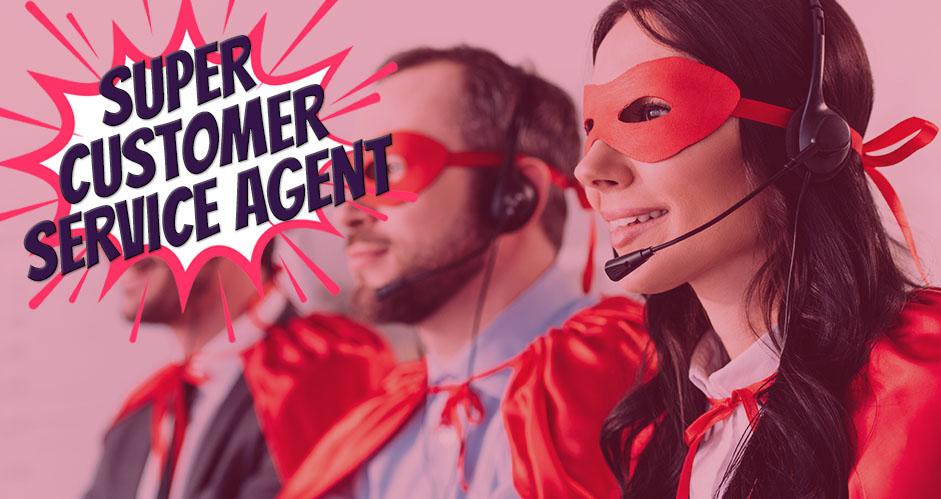
Predictive Customer Profiling and Personalized Recommendations
LLMs can analyze customer behavior, interaction history, and feedback to create comprehensive customer profiles. Based on these profiles, LLMs can predict customer needs and preferences, and proactively offer personalized solutions, product recommendations, or support. This predictive profiling and personalization can lead to improved customer satisfaction and loyalty, giving businesses a competitive edge.
Customer profiling and recommendation required lots of data and resources to implement correctly. This is usually not accessible to smaller businesses, or businesses that are just starting. LLMs make this available for everyone, at a low cost.
Sentiment Analysis and Feedback Interpretation
LLMs can parse through customer feedback, reviews, social media comments, and more to analyze sentiment and understand customer perceptions about a product, service, or overall brand. By identifying trends, pinpointing issues, and understanding customer sentiment, businesses can make data-driven decisions to improve their offerings and customer service. Moreover, they can also identify potential advocates or detractors, helping them create targeted engagement strategies.
Employee training
ChatGPT (like applications) can also be used to train customer support staff. This kind of tech can help employees provide better answers and respond faster to customer queries. Furthermore, new employees can start being productive much faster. This also helps at Peak Times or even when staff are short, as less qualified employees can be temporarily deployed.
Incorporating LLMs into customer service processes allows businesses to provide superior, personalized customer experiences at scale, and gain valuable insights into their customer base. Whether by improving existing processes or introducing AI capabilities from scratch, LLMs offer a significant leap in quality compared to traditional NLP methods.
Hallucinations in Large Language Models
LLMs can sometimes generate incorrect or contextually inappropriate text, a phenomenon known as "hallucination." This can be a problem in the context of customer service or any customer facing scenario.
Hallucination can stem from various factors:
- Insufficient Training Data: Lack of diverse and comprehensive data can lead to fabricated information.
- Biases and Prejudices: Models might reflect societal biases present in the training data.
- Contextual Ambiguity: Ambiguity in context can lead to incorrect interpretations.
- Knowledge Gaps: Lack of information might cause the model to generate fictional details.
- Adversarial Attacks: Malicious inputs can deceive the model into generating incorrect outputs.
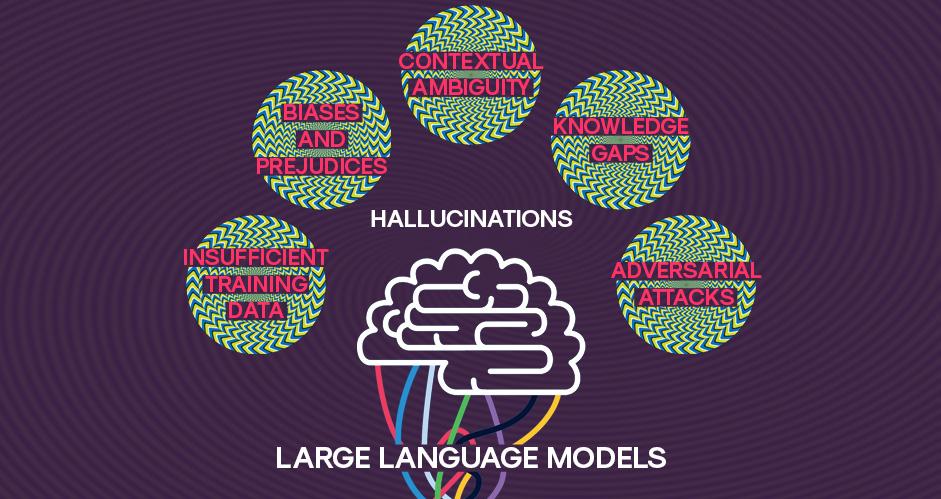
Hallucination has significant implications, especially in critical domains like healthcare, finance, and legal. Careful validation and ongoing monitoring are vital to maintain trust and reliability. In a customer facing scenario, hallucinations can lead to incorrect, nonsensical, inappropriate, harmful or offensive output. This could damage a brand, and lead to loss of customers and credibility.
Researchers are working on strategies like data augmentation, debiasing algorithms, improved context representation, external knowledge integration, and robust defense mechanisms to minimize hallucinations, as well as determining attribution of Language Model output like this work from Google.
The research community is focused on improving training methodologies, reducing biases, handling adversarial attacks, and ensuring ethical use. Balancing creativity with accuracy and addressing the risks associated with hallucination is vital for the future of reliable and trustworthy language models.
The continuous evolution of large language models offers exciting prospects but also presents challenges. Ensuring accuracy, transparency, and responsibility in these models is key to harnessing their potential without losing credibility.
Implementing LLMs in Customer Service: How to get Started
Implementing LLMs into your customer service processes requires careful consideration. It starts with understanding your business needs and identifying the areas where LLMs can bring the most value.
Furthermore, it's important to remember that LLMs, while impressive, are not yet perfect. They can sometimes produce outputs that are incorrect, nonsensical, or even inappropriate. Hence, careful monitoring and fine-tuning are required to ensure these models perform effectively and ethically.
So, where to start?
The safest way to start is in scenarios that are not customer facing, but still bring value by enhancing productivity, quality of answers to customers, but still include a human in the loop, before sending any information to customers.
Because LLMs can produce unpredictable answers, it's important to make sure that whatever is sent to a customer, is attributable to some source of truth, and can be traced back to it. This is important for credibility.
And it probably goes without saying that having the right professionals handling the implementation is a huge plus. If you are interested in taking that journey and getting your customer service to the next level, we at Two Impulse would be honored to partner with you throughout the whole process. Just get in touch with us here.
The Potential of LLMs in Customer Service
The potential of Large Language Models in customer service is immense, offering a leap in quality and efficiency that far surpasses traditional methods. As businesses seek to elevate their customer service, LLMs can provide a path towards delivering superior, personalized customer experiences.
However, to truly harness the power of this technology, businesses need to navigate its implementation and use with care and responsibility, ensuring they deliver value to the customers while safeguarding their data and privacy, while making sure not to lose credibility in the process.